Exploración de los factores que influyen en la integración de herramientas de dibujo en la educación en artes y diseño
Palabras clave:
Herramientas de dibujo de inteligencia artificial, educación en arte y diseño, efectos de la aplicación, deficiencias y contramedidaResumen
El propósito de este estudio es explorar los factores que influyen en la integración del uso de herramientas de dibujo de inteligencia artificial en la educación de arte y diseño en colegios y universidades. Para integrar la tecnología de IA en la educación de diseño de arte, primero es necesario aclarar el efecto de la aplicación de la tecnología de IA en el proceso educativo. A través de los métodos de revisión de literatura, estudio de casos y enseñanza práctica, analizamos el potencial y los factores influyentes de la aplicación de IA en la educación mediante la integración de los fundamentos teóricos de la enseñanza de arte y diseño con IA. Este estudio resume los efectos y las posibles deficiencias de la aplicación de la inteligencia artificial a partir del caso de las universidades en China y propone contramedidas correspondientes. Las conclusiones del estudio pueden proporcionar apoyo teórico y orientación práctica para la aplicación de la inteligencia artificial en la enseñanza del arte y el diseño.Citas
Li, S. (2019). The impact of artificial intelligence on design education. Art Education, 6, 261.
Yang, Z., Wang, J., Wu, D., & Chen, X. (2023). Exploring the impact of ChatGPT/AIGC on education and strategies for response. Journal of East China Normal University, Educational Sciences, (7), 26.
Yang, Y. (2022). Discussion on the development and application of artificial intelligence technology in the field of art and design. Culture and Art Innovation-International Academic Forum, 2(5), 79.
Atapattu, T., Falkner, K., & Thilakaratne, M. (2020). What do linguistic expressions tell us about learners’ confusion? A domain-independent analysis in MOOCs. IEEE Transactions on Learning Technologies, 13(4), 878–888.
Beseiso, M., Alzubi, O. A., & Rashaideh, H. (2021). A novel automated essay scoring approach for reliable higher educational assessments. Journal of Computing in Higher Education, 33, 727–746.
Donthu, N., Kumar, S., & Mukherjee, D. (2021). How to conduct a bibliometric analysis: An overview and guidelines. Journal of Business Research, 133, 285–296.
Dutt, S., Ahuja, N. J., & Kumar, M. (2022). An intelligent tutoring system architecture based on fuzzy neural network (FNN) for special education of learning disabled learners. Education and Information Technologies, 27, 2613–2633.
Chen, L., Chen, P., & Lin, Z. (2020). Artificial intelligence in education: A review. IEEE Access, 8, 75264–75278.
Yu, Z., & Yuan, W. (2021). Research on design education based on artificial intelligence technology. Creativity and Design, (74), 86.
Huang, A. Y., Lu, O. H., & Huang, J. C., et al. (2020). Predicting students’ academic performance by using educational big data and learning analytics: Evaluation of classification methods and learning logs. Interactive Learning Environments, 28(2), 206–230.
Hwang, G. J., & Tu, Y. F. (2021). Roles and research trends of artificial intelligence in mathematics education: A bibliometric mapping analysis and systematic review. Mathematics, 9(6), 584.
Lee, C. A., Tzeng, J. W., & Huang, N. F., et al. (2021). Prediction of student performance in massive open online courses using deep learning systems based on learning behaviors. Educational Technology & Society, 24(3), 130–146.
Lin, C. J., & Mubarok, H. (2021). Learning analytics for investigating the mind map-guided AI chatbot
approach in an EFL flipped speaking classroom. Educational Technology & Society, 24(4), 16–35.
Roll, I., Aleven, V., & McLaren, B. M., et al. (2011). Improving students’ help-seeking skills using metacognitive feedback in an intelligent tutoring system. Learning and Instruction, 21(2), 267–280.
Sinclair, J., Jang, E. E., & Rudzicz, F. (2021). Using machine learning to predict children’s reading comprehension from linguistic features extracted from speech and writing. Journal of Educational Psychology, 113(6), 1088–1106.
Tong, L., Zhang, C., Huang, R., Lei, F., & Yang, Z. (2022). Educational social experiment: A new exploration of the integration of artificial intelligence into education. China Electrification Education, 422, 64.
Nasseri, M. (2021). Is postgraduate English academic writing more clausal or phrasal? Syntactic complexification at the crossroads of genre, proficiency, and statistical modelling. Journal of English for Academic Purposes, 49, 100940.
Urteaga, I., Siri, L., & Garofalo, G. (2020). Early dropout prediction via machine learning in professional online courses. RIED. Revista Iberoamericana de Educación a Distancia, 23(2), 147-167.
Van Eck, N. J., & Waltman, L. (2010). Software survey: VOSviewer, a computer program for bibliometric mapping. Scientometrics, 84(2), 523-538.
Wang, D., Han, H., Zhan, Z., et al. (2015). A problem-solving oriented intelligent tutoring system to improve students’ acquisition of basic computer skills. Computers & Education, 81, 102-112.
Wu, P. H., Hwang, G. J., & Tsai, W. H. (2013). An expert system-based context-aware ubiquitous learning approach for conducting science learning activities. Journal of Educational Technology & Society, 16(4), 217-230.
Qin, C. (2020). Application of artificial intelligence in art and design education. Computer Paradise, 400.
Zhai, X., Shi, L., & Nehm, R. H. (2021). A meta-analysis of machine learning-based science assessments: Factors impacting machine-human score agreements. Journal of Science Education and Technology, 30, 361-379.
Yang, Y. (2022). Discussion on the development and application of artificial intelligence technology in the field of art and design. Culture and Art Innovation-International Academic Forum, 2(5), November.
Zhu, M., Liu, O. L., & Lee, H. S. (2020). The effect of automated feedback on revision behavior and learning gains in formative assessment of scientific argument writing. Computers & Education, 143, 103668.
Hwang, G., Fang, J., & Tu, Y. (2022). International research prospects and trends of artificial intelligence in education. Modern Distance Education Research, 34(3).
Agrawal, A. (2018). Application of machine learning to computer graphics. IEEE Computer Graphics and Applications, 93.
Raffel, C., Shazeer, N., Roberts, A., Lee, K., Narang, S., Matena, M., Zhou, Y., Li, W., & Liu, P. (2019). Exploring the limits of transfer learning with a unified text-to-text transformer. Journal of Machine Learning Research, 21, 1-67.
Wei, J., Wang, X., Schuurmans, D., Bosma, M., Chi, E., Le, Q., & Zhou, D. (2022). Chain-of-thought prompting elicits reasoning in large language models. Advances in Neural Information Processing Systems, 36.
Li, Z., Zhou, D., Liu, N., & Ou, Y. (2018). Research on the artificial intelligence in education under the background of artificial intelligence application. Modern Educational Technology, 28(9).
Roy, S., & Dan, R. (2015). Solving general arithmetic word problems. In Proceedings of the Conference on Empirical Methods in Natural Language Processing (pp. 1743-1752).
Adams, C., Pente, P., Lemermeyer, G., & Rockwell, G. (2023). Ethical principles for artificial intelligence in K-12 education. Computers and Education: Artificial Intelligence, 100131.
Yan, H., Liu, Y., Jin, L., & Bai, X. (2023). The development, application, and future of LLM similar to ChatGPT. Journal of Image and Graphics, 28(9).
Tay, Y., Dehghani, M., Tran, V. Q., Garcia, X., Wei, J., Wang, X. Z., Chung, H. W., Bahri, D., Schuster, T., Zheng, H. S., Zhou, D., Houlsby, N., & Metzler, D. (2023). UL2: Unifying language learning paradigms. In Proceedings of the Eleventh International Conference on Learning Representations (pp. 1-33). Kigali, Rwanda: OpenReview.
Yang, Z., Wang, J., Wu, J., & Chen, X. (2023). The impact of ChatGPT/generative artificial intelligence on education and response strategies. Journal of East China Normal University, Educational Sciences, 7.
Devlin, J., Chang, M. W., Lee, K., & Toutanova, K. (2019). BERT: Pre-training of deep bidirectional transformers for language understanding. In Proceedings of the 2019 Conference of the North American Chapter of the Association for Computational Linguistics (pp. 4171-4186). Minneapolis, USA: Association for Computational Linguistics. https://doi.org/10.18653/v1/N19-1423
Deng, J., Dong, W., Socher, R., Li, L., Li, K., & Fei-Fei, L. (2009). ImageNet: A large-scale hierarchical image database. In Computer Vision and Pattern Recognition, 2009. CVPR 2009. IEEE Conference on (pp. 248-255). IEEE.
Hoffer, E., & Ailon, N. (2015). Deep metric learning using triplet network. In International Workshop on Similarity-Based Pattern Recognition (pp. 84-92). Springer.
Mao, H., Cheung, M., & She, J. (2017). Engagement 2 - Digital Society & Multimedia Art, Entertainment and Culture. In MM '17: ACM Multimedia Conference (pp. 1-6). Mountain View, CA: ACM.
Li, J., & Wang, J. Z. (2004). Studying digital imagery of ancient paintings by mixtures of stochastic models. IEEE Transactions on Image Processing, 13(3), 340-353.
Quan, G., Gu, X., & Wang, J. (2021). Research on the "enabling" effect of visual interaction in artificial intelligence education application. Open Education Research, 27(4), 111.
Manjunath, B. S., & Ma, W. Y. (1996). Texture features for browsing and retrieval of image data. IEEE Transactions on Pattern Analysis and Machine Intelligence, 18(8), 837-842.
Luckin, R., & Holmes, W. (2022). Intelligence unleashed: An argument for AI in Education. ResearchGate. https://www.researchgate.net/publication/303014375_Intelligence_Unleashed_An_Argument_for_AI_in_Education
Sdenka, Z., & Salas, P. (2020). The impact of AI and robotics on physical, social-emotional, and intellectual learning outcomes: An integrated analytical framework. British Journal of Educational Technology, 51, 1808-1825.
McCarthy, J., Minsky, M. L., et al. (2006). A proposal for the Dartmouth Summer Research Project on Artificial Intelligence. AI Magazine, 4, 1-13.
Mao, H., Cheung, M., & She, J. (2017). Engagement 2 - Digital Society & Multimedia Art, Entertainment and Culture. In Proceedings of the 2017 ACM Multimedia Conference (pp. 1-6). Mountain View, CA: ACM.
Russell, S., & Norvig, P. (2009). Artificial Intelligence: A Modern Approach (3rd ed.). Upper Saddle River: Prentice Hall.
Rahwan, I., Cebrian, M., et al. (2019). Machine behaviour. Nature, 7753, 477-486.
Dunn, K. E., & Mulvenon, S. W. (2009). A critical review of research on formative assessment: The limited scientific evidence of the impact of formative assessment in education. Practical Assessment Research & Evaluation, 14(11).
Zeng, Y., & Wang, L. (2017). Artificial intelligence is on its way to reshape the world. National Science Review, 3, 490-492.
Crawford, K., & Calo, R. (2016). There is a blind spot in AI research. Nature, 538(7625), 311-313.
Perry, J., & Perry, E. (2015). Contemporary society: An introduction to social science. New York: Routledge.
Mohr, Z. T. (2019). Experiments in public management research: Challenges and contributions. Public Administration Review, 79(2), 286-289.
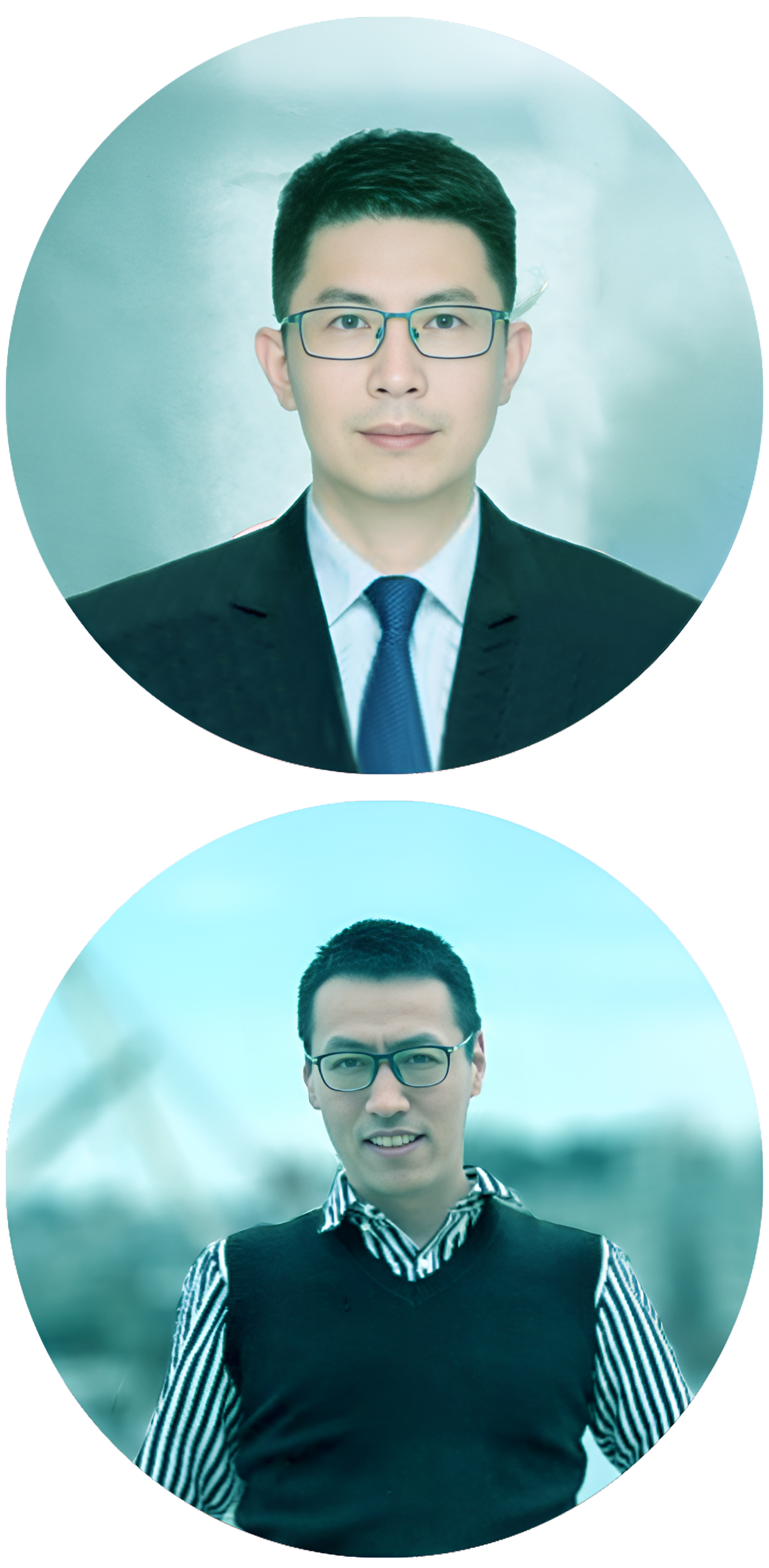
Publicado
Cómo citar
Número
Sección
Licencia

Esta obra está bajo una licencia internacional Creative Commons Atribución 4.0.
Usted es libre de:
- Compartir — copiar y redistribuir el material en cualquier medio o formato
- Adaptar — remezclar, transformar y crear a partir del material
- para cualquier finalidad, incluso comercial.
- Reconocimiento — Debe reconocer adecuadamente la autoría, proporcionar un enlace a la licencia e indicar si se han realizado cambios<. Puede hacerlo de cualquier manera razonable, pero no de una manera que sugiera que tiene el apoyo del licenciador o lo recibe por el uso que hace.
- No hay restricciones adicionales — No puede aplicar términos legales o medidas tecnológicas que legalmente restrinjan realizar aquello que la licencia permite.